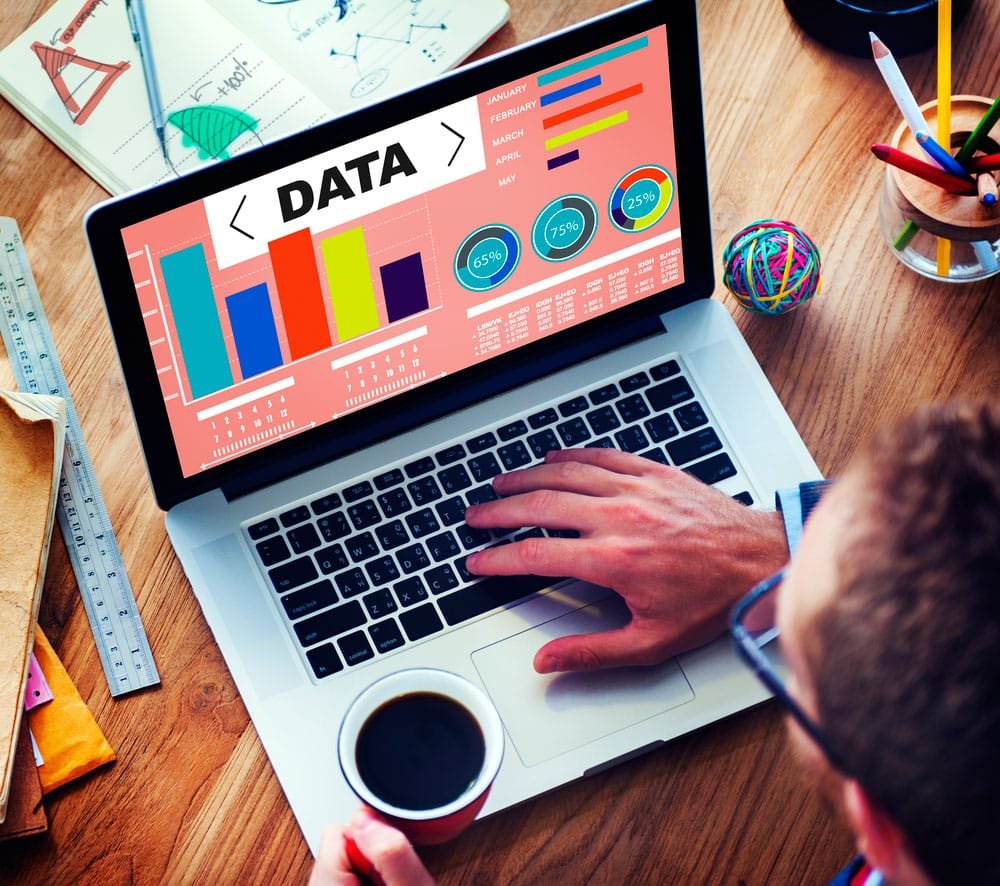
So far, the year of 2022 has been pointing in the direction of data being fused with software engineering for data exploration, data quality monitoring and observability, specialization of different ETL layers, and data security; these are all areas that thrived in 2021.
Whether startup companies or billion-dollar corporations continue to store and process data into databases, cloud data warehouses, data lakes, and data lakehouses.
Let’s dig into the trends that you should know about the modern data stack going into 2022.
Data Mesh
The core idea behind data mesh is that companies can become more data-driven by going from centralized data warehouses and lakes to a domain-oriented decentralized data ownership and architecture driven by self-serve data and federated computational governance.
To clarify, data mesh isn’t a particular service or platform that you can purchase off the shelf. It can be described as a design concept with some wonderful concepts like distributed ownership, domain-based design, data discoverability, and data product shipping standards — all of which are worth trying to operationalize in your organization.
Reverse ETL
Reverse ETL syncs up data from a system of records like a data warehouse to a system of actions like CRM and MAP (SaaS apps) to operationalize data.
Basically, when you are talking about the 2022 data stack trend of reverse ETL, you are referring to the process that lets you transport data about your users from your warehouse and makes it available for frontline business teams to use with the digital tools that they are familiar with.
To really wrap your mind around the power of reverse ETL and to get to the bottom of why it’s not just another data pipeline, look no further than what traditional ETL pipelines have made possible for business and data teams.
The normal Extract, Transform, Load (ETL) data pipeline has stayed largely the same since the 1970s; pull data from the source, convert it to a usable format, then send it into your data warehouse.
Now with flexible data pipeline tools on the horizon, you can load your data into the data warehouse and then use your storage target to transform it (referred to as ELT).
In fact, these ETL/ELT have let companies combine data from multiple sources into one singular single source of truth in order for business decisions to be made based on facts instead of assumptions.
Data Teams as Product Teams
The modern data stack is becoming much more mainstream; all the while, data is becoming a larger part of daily operations, raising the question of how data teams should work with the rest of their company.
The importance of the role they play is expanding beyond the service sector questions and requests for creating stats and are now generating data insights and driving impact.
Another trend we are seeing in 2022 is data product teams being more centered on the users (data consumers throughout the organization) instead of questions answered or dashboards built.
Data teams are focusing more on the user experience, adoption, and reusability.
This reorientation of data teams around their users really resonated with the data world, with more organizations building data product teams.
Demand for Analytics Engineers
The need for data engineers grew in 2020 and 2021. But in 2022, we’re all about the rise of the data engineer (which, according to Dice’s tech jobs report, was the fastest-growing tech job) in 2022; the analytics engineer will make its definitive entrance into the spotlight.
The rise of the role that analytic engineers are playing can be attributed to the rise of cloud data platforms and data build tools (dbt). Dbt labs, the company that is known for bringing out dbt, actually coined the role of analytic engineer.
An increasing number of modern data teams are adding analytics engineers to their teams as they’re becoming more and more data-driven and building self-serve data pipelines.
Metrics Layers
Metrics are key to sizing up and driving a company’s growth, but they’ve often been split across various data tools, with different definitions for the same metric across different teams or dashboards.
But in 2021, people started talking publicly, discussing how the modern data stack could fix this issue.
The growing solution in the space is the concept of a metrics layer (other terms for this are headless BI or metrics store).
The metrics layer can be that central, single source of truth for how metrics are defined in your organization, between where your data is stored and how it is consumed for a unified definition.
Your organization has multiple dashboards, and it may also have multiple business intelligence (BI) tools.
Therefore, do you want to waste time having to define the business logic for your metrics each time in each of those outlets, especially if the logic changes with the growth of your business?
But a single agreed-upon definition that is used in multiple places solves this problem, making it a data stack trending for 2022.
ATTENTION READERS
We See The World From All Sides and Want YOU To Be Fully InformedIn fact, intentional disinformation is a disgraceful scourge in media today. So to assuage any possible errant incorrect information posted herein, we strongly encourage you to seek corroboration from other non-VT sources before forming an educated opinion.
About VT - Policies & Disclosures - Comment Policy